FORT BRAGG, North Carolina –
As the military transitions its focus to large-scale combat operations (LSCO) within the context of the multi-domain doctrinal concept, significant challenges facing the military health system were identified for which there are no immediate solutions.
01,02 Alongside this shift in focus, strategic documents and senior military leaders suggest that ubiquitous data collection, robust cyber-secure networks, massive processing power, and scalable artificial intelligence (AI) constitute a technological revolution that is changing the character of war.
03 The military health system’s ability to deliver tactical combat casualty care must evolve along with doctrinal and technological changes. The military health system needs to seize the opportunity to rapidly shift its priorities and resources to address these changes. This article helps address the imminent yet unwritten requirement to apply emerging technologies to military medical training.
Through passive data collection, robust data analysis, and future modeling with AI tools, the Army can accelerate an individual’s acquisition and sustainment of essential medical knowledge, skills, and abilities by applying
digital twins to military medical training. The term digital twins, for the context of this article, refers to the virtual representation of physical objects, processes, or systems. We introduce the concept of
humanoid digital twins as a construct to predict the personalized training needs of individual soldiers to improve the ready medical force. The article highlights a use case named
Measuring and Advancing Soldier Tactical Readiness and Effectiveness (MASTER-E) program, similar to professional sports activities, as exemplars of data-driven personalization of training and readiness. Finally, we emphasize some significant challenges within modernization and innovation regarding this emerging technology.
CURRENT MILITARY MEDICAL TRAINING
To adequately prepare a ready medical force for the future fight, it is imperative that the military health system conducts realistic training to address challenges identified by threat-based and scenario-driven assessments. Training should improve combat medical performance under tactically relevant stressors, but is today’s training effective? Current military medical training focuses heavily on the acquisition and sustainment of individual knowledge, skills, and abilities based on programs of instruction. However, the delivery of most tactical combat casualty care is within a system-of-systems: a care continuum from the point of injury through Role 4, including casualty evacuation, medical evacuation, and strategic evacuation, which also involves collective team performance.
The military health system has minimal training environments to test this care continuum under tactically relevant stressors. Specifically, limitations exist in testing the system’s ability to manage casualties due to the lack of casualty and contextual realism and medical decision-making during large-scale exercises. Medical decision-making is complex, nuanced, and dependent on resource availability, environment, and mission.
04
Without realistic casualties, sufficient medical supplies, and realistic casualty outcomes within a realistic care context, medical decision-making during training may not reflect actual medical decision-making during combat. While individual training remains essential, future success depends on improving and continuous assessments of the care continuum and (individual and collective) team performance. A continually measured performance-based learning ecosystem will enable data driven models to review and reassess outcome performance metrics over time.
Furthermore, training centers often rely on manikins that may not fully capture the entirety of the casualty care experience, are costly and time-consuming to maintain, and require highly trained personnel to utilize the equipment. Current manikins lack modularity, interoperability, scalability, and sensor data feedback with appropriate automated physiologic responses to medical interventions.
Lastly, systematic and personal bias may exist depending on the training environment and instructors’ experience. Instructors may be unfamiliar with tactical combat casualty care under actual circumstances or lack knowledge about physiology, injury progression, or treatment side effects. This contributes to sub-standard and insufficient feedback for trainees regarding critical thinking and decision-making under realistic operational training environments.
DIGITAL TWINS AND ARTIFICIAL INTELLIGENCE
Today, digital twin technology is more prevalent within modeling and simulation (M&S). According to the Government Accounting Office, a digital twin is defined as a “virtual representation of physical objects, processes, or systems—like factories, traffic patterns, and even people…to predict how changes may affect its physical counterpart.”
05
Some common-use examples of data twin technology have become routine for the everyday user as the data architecture is well established. To improve efficiency and effectiveness in planning and directing trips on the road, GPS mapping companies use modeling to optimize traffic flow for individual users.
06 Package delivery companies update deliveries based on new orders, delivery vehicle availability, delivery addresses, and traffic patterns in real-time based on sensor input distributed across the supply chain.
07
To understand digital twins, it is crucial to comprehend “deep learning” and “neural networks.” Deep learning is a method to train AI systems composed of neural networks, a method in artificial intelligence (AI) that teaches computers to process data in a way inspired by the human brain to impute knowledge from large datasets.
08 In this methodology, multiple computational “layers” process complex, high-dimensional data iteratively to identify meaningful patterns within the data. In this process, instead of a software programmer producing a series of “if-then” statements to help a computer recognize <chairs> by identifying legs, a back, and a horizontal surface for sitting, a data scientist provides thousands-to-millions of images labeled as <chairs> and a similar dataset labeled as <not chairs> and allows the computer to segment the images according to pixel patterns to identify a pattern that predicts <chairs> accurately.
Typically, an additional dataset of <chairs> and <not chairs> is used to validate the neural network’s predictions. Where predictions are incorrect, the model can be refined and reprocessed. In supervised (using labeled datasets and human-derived rules) and unsupervised (computer-generated pattern recognition only) machine learning, selecting the best models to fit available datasets and validating model outputs is essential.
09 Table 1 provides terms, definitions, and concepts related to data, modeling and simulation, AI, and machine learning.
APPLYING DIGITAL TWINS TO CASUALTY CARE AND HUMAN PERFORMANCE
An example of an Army application regarding humanoid digital twins involves a complete simulation of the internal anatomy of the human body. The humanoid digital twin is fully articulated, animated, and possesses simulated physiology and biomechanics. A humanoid digital twin with complete internal anatomy was introduced in the last decade to provide an initial Army test bed for military performance research.
10 Comparison of the humanoid digital twin's monitored physiology data against experimental protocols has shown a high degree of correlation.
11 More importantly, humanoid digital twins can be tasked to perform in extreme environments of temperature, altitude, and other environmentally hazardous circumstances in a virtual world that is safe and replicable based on external and internal inputs.
Training with a virtual representation of a live Soldier can result in human performance improvements comparable to professional athletes. According to the Director of Performance Innovation Business Operations at the United States Olympic and Paralympic Committee, Mike Levine, “Utilizing advances in AI and computer vision, we've been able to track and study personalized analytics from a variety of sports to determine the strengths and deficiencies in an athlete's movement and help them make data-informed training and competition plans that can help them improve their performance, as well as their own health."
12 These technologies can provide a foundation for a comprehensive understanding of human (individual and collective team) performance and enhance decision-making through AI-driven optimization by leveraging incremental improvements via micro-learning tasks that are targeted and personalized to the user.
We present a use case called the
Measuring and Advancing Soldier Tactical Readiness and Effectiveness (MASTR-E) Program. Currently, this is the largest human performance science and technology program within the U.S. military. Similar to professional sports teams, it creates quantifiable metrics by sensing human physiology (through the use of wearable sensors) to forecast performance (Figure 1).
13,14,15
Figure 1. MASTR-E predictors of performance (social-emotional, health, physical, and cognitive) with constructs specific to Measures of Performance (MOPs) and Measures of Effectiveness (MOEs).
This modeling approach will be applied to the other operational domains critical to Soldier performance, including Move, Sustain, Navigate, and Communication (Figure 1). Future directions of this work include a focus on the degradation of the Soldier over time and measures of lethality, identifying unique combinations of features that better classify sub-populations, defining aggregated domain scores (physical, health, socio-emotional, cognitive), and prediction-constrained factor analysis for interpretable predictions of Soldier performance.
This model, which amounts to a humanoid digital twin of Soldiers performing small unit non-medical tasks, is translated into a “small unit dashboard” that displays relative performance metrics for leader decision points. In addition to modeling individual and collective team performance reasonably, humanoid digital twins must support leaders' decision-making and readiness postures. For this reason, it is critical to continue developing predictive performance models that can impact the military decision-making process and include data driven dashboards that improve Soldiers’ readiness. Dashboards created from these data ecosystems allow commanders to review a snapshot of their unit readiness. This allows for improved risk assessments and training plans before missions.
Successful integration of humanoid digital twin models measuring individual and collective team performance (in live, virtual, and constructive domains) will optimize unit performance prior to deployment cycles. Additionally, a suite of capabilities that sense, analyze, display, and precisely model performance using humanoid digital twins will reduce training time and increase Soldier competency. As a result, this will provide a smarter, faster, and more precise learning ecosystem for Soldiers and leaders at all echelons. Developing humanoid digital twin models requires AI and machine learning and an adequate focus on Soldier readiness. However, the existing digital infrastructure of the military health system is neither sufficient nor targeted toward collecting, storing, analyzing, and curating data for modeling humanoid digital twins.
CURRENT CHALLENGES
When discussing humanoid digital twins and precision training, the rich data layer that describes the individual and collective team performance—necessary to produce meaningful models—is often overlooked. This data layer is derived from passive data collection of human performance when executing individual and collective tasks. Further, the data must be programmed to contain meaningful labels derived from human experience prior to leveraging humanoid digital twin models to inform improved decisions. Applicable models, methods, and metrics relevant to generating and labeling this data are still nascent, especially regarding medical performance optimization and readiness. There are few trainings and no real-world military medical environments that collect data about caregiver performance using passive sensors and store that data for later modeling.
For example, the MASTR-E advanced, data-driven proficiency assessment and after action review system utilizes cutting-edge performance analytics to measure, assess, and portray tactical training outcomes at the individual and small unit levels.
12 This concept is similar to the humanoid digital twin of an athlete whose coach can target training based on data-driven metrics for optimal performance on the athletic field (Figure 2).
Figure 2. MASTR-E small unit performance analytics with effective individual outcome metrics for data-driven decisions.
In other words, the system allows Soldiers and units to target individual deficiency patterns and success to optimize training outcomes. The medical community could adopt a comprehensive training approach using objective metrics and subjective feedback from observers, coaches, and trainers. This approach could be applied in both live and simulated training environments, generating a large dataset of performance information that can be used to create personalized, digital replicas of individuals to optimize training and improve readiness. Medical human performance modeling and simulation within research and training environments have essential components that include:
- Tracking and visualization of measures of performance and measures of effectiveness during individual live-fire and simulated proficiency assessments performed under tactically relevant stressors.
- Automated/digitized after action review platform for validated performance quantification and visualization of small unit performance during battle drill execution, to include automated cueing of deficiencies for targeted training (“shoot, move, communicate, and medicate”).
- Commitment to a training integration plan for implementing modeling and simulation within the training cycle.
- Measures of performance and measures of effectiveness that are easily collected for visualization and review provide Soldiers and leaders the ability to quantify deficiencies and strengths, and train deliberately.
- A core dataset that can be collected in research, training, and real-world casualty care environments across the entire care continuum (Point of Injury through Role 4).
While it is imperative to emphasize the models, methods, and metrics that can produce the core dataset of human performance, it is equally important to define the requirements for governance and data architecture to collect, store, analyze, portray, and model within the data pipeline.
16
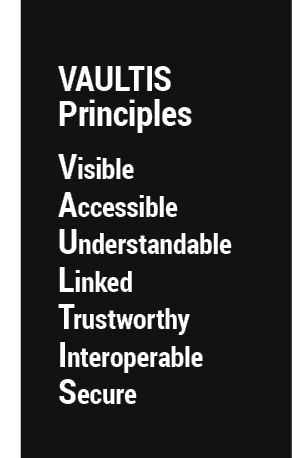
The Fiscal Year 2024 Army AI Data Strategic Action Plan,
9 states all data and modeling efforts must adhere to the VAULTIS principles wherein data and models are Visible (V), Accessible (A), Understandable (U), Linked (L), Trustworthy (T), Interoperable (I), and Secure (S). This requires seamless communication, coordination, and safeguarding of data and software from the lowest to the highest echelon of oversight. Data architecture must also adhere to a Modular Open Systems Approach per Title 10 United States Code, Section 805, 2446a.(b). The DoD will deploy modular design interfaces between major system platforms and ensure that there are government rights to the tech stack, which is integral to the engineering requirements for product development.
17 Moreover, it's imperative for the organizational culture to set realistic technology goals. Being realistic involves harmonizing new systems with existing legacy systems and investing in the development of a skilled workforce fully trained in these technologies. The end state would be to achieve more relevant, cost effective, and time efficient performance goals and outcomes.
18 Other substantial challenges to developing humanoid digital twins are highlighted in Table 2.
According to a report from the Director of Operational Test and Evaluation, the digital twins' verification, validation, and accreditation process is still under development. Guidance from the Director of Test and Evaluation, in coordination with the Office of the Under Secretary of Defense for Research and Engineering, indicates that the interim process for verification and validation of modeling and simulation will apply to digital twins. The report further asserts that the plan for the use of digital twins with commercial virtualization technologies within DoD must include the following:
17
- Requirement “to assess the dependencies of the system and its components on specialized real-time computer processing wherein virtualization may pose a performance or power consumption concern for tactical applications”16
- Requirement to evaluate the benefits of commercial virtualization (e.g., Virtual Machines, Hypervisors, Docker Containers, and Kubernetes Clusters), “including energy savings, potential reliability improvements, and hardware savings while creating and maintaining more complex security boundaries”17
- Commitment to develop “tools and processes needed to adequately evaluate the operational performance of systems employing such technologies”17
Finally, a lack of a data strategy, data standards, interoperability framework, and infrastructure for data storage within the MHS are significant barriers to successfully developing AI medical assessment tools to equip the ready medical force. These foundational components of an AI learning ecosystem must be created specifically for the medical community, especially when patient data is incorporated into the training model. Developing these capabilities will allow the medical science and technology (S&T) community to build and refine individual and collective performance models. As these models mature, they can be integrated into more precise humanoid digital twins of the trainee, the instructor, and the tactical combat casualty care delivery ecosystem by fusing past and current data for trend analyses and future predictive modeling.
While MASTR-E developed individual Soldier and small unit performance data of non-medical tasks, there does not appear to be similar development efforts for model and simulation in the MHS. However, there are nominal efforts within the Medical Research and Development Command and the Telemedicine and Advanced Technology Research Center. Failure to modernize training data and analyses may result in significant readiness gaps and contribute to cognitive and physical performance deficiencies. Consequently, these critical gaps also impact readiness to perform effectively during the most critical moments of combat casualty care.
FUTURE IN TRAINING
In the future, training that translates to Soldier readiness will require a well-governed data architecture and learning ecosystem that can leverage emerging technologies like AI for more focused performance outcomes. Holistically, the current evaluations of technical skills need more objective and consistent metrics of success. Non-technical skills such as stress management and teamwork are often indirectly addressed through instructor feedback rather than objective and reliable debriefing from all perspectives.
19 Limitations on
time imposed by instructors and limited user
trust in simulation and training are magnified by budget and resource constraints, directly affecting the continual learning ecosystem. Therefore, digital twins are necessary for maximizing limited training time while increasing trainee
trust, leading to a more ready medical force.
Integrated with a common data architecture to optimize performance in live, virtual, and constructive training domains, these humanoid digital twins will utilize objective performance analytics. Analytics would then measure, assess, and portray tactical performance and effectiveness measures for improved training outcomes within a digital learning ecosystem. Furthermore, non-traditional applications of humanoid digital twins will likely appear as new technology emerges. For example, augmented reality or mixed reality of the humanoid digital twin’s anatomy can be superimposed upon a live person or object. Future applications could include using injured Soldiers during battlefield triage and utilizing the Integrated Visual Augmented System-based software tool.
20,21
The future operational environment remains complex and uncertain. The ever-changing manifestation of great power competition may prove unpredictable as irregular warfare tactics only add to the volatility within battlefield operations. Optimizing operational medical readiness and improved training outcomes will require rapid innovation, partnerships for programs and policies, plus sound and sustainable solutions for well-defined and validated problems. Humanoid digital twin technology provides a solution to deliver personalized and dynamic training at a scale that ensures a ready medical force to improve the survivability and lethality of the Soldier.
Editor’s Note: Clarification for the reader, in the “Digital Twins and AI section,” narrative was written using the greater than and less than symbols <chairs>. This is an example of computer programming language; it is AI labeling and can substitute any object.
REFERENCES
01 Remondelli MH, Remick KN, Shackelford SA, Gurney JM, Pamplin JC, Polk TM, Potter BK, Holt DB. Casualty care implications of large-scale combat operations. Journal of Trauma and Acute Care Surgery. 2023 Aug 1;95(2S):S180-4.
02 Epstein A, Lim R, Johannigman J, Fox CJ, Inaba K, Vercruysse GA, Thomas RW, Martin MJ, Konstantyn G, Schwaitzberg SD. Putting medical boots on the ground: lessons from the war in Ukraine and applications for future conflict with near-peer adversaries. Journal of the American College of Surgeons. 2023 Aug 1;237(2):364-73.
03 Milley MA. Strategic Inflection Point: The Most Historically Significant and Fundamental Change in the Character of War Is Happening Now—While the Future Is Clouded in Mist and Uncertainty. Joint Force Quarterly. 2023 Jul;110(9).
04 Croskerry P. Achieving quality in clinical decision making: cognitive strategies and detection of bias. Academic emergency medicine. 2002 Nov;9(11):1184-204.
05 Science & Tech Spotlight: Digital Twins—Virtual Models of People and Objects. US Government Accountability Office. https://www.gao.gov/products/gao-23-106453 Published February 14, 2023.
06 West, Josie [@Josie_West]. 18 August 2021 “Is Google Maps a Digital Twin?” LinkedIn. Accessed 3 March 2024 at https://www.linkedin.com/pulse/google-maps-digital-twin-josie-west
07 Bolle S, Foiz P, Ramparany F, Zijp Rouzier S, Coupaye T. “More Sustainable Urban Logistics Using Digital Twins.” Hello Future Research Blog. 2 November 2023. Accessed 15 March 2024 at https://hellofuture.orange.com/en/more-sustainable-urban-logistics-using-digital-twins/
08 Department of Defense - Data, Analytics, Artificial Intelligence Adoption Strategy: Accelerating Decision Advantage. Washington, DC: Dept of Def; 2023. https://media.defense.gov/2023/Nov/02/2003333300/-1/-1/1/DOD_DATA_ANALYTICS_AI_ADOPTION_STRATEGY.PDF
09 Zientara GP, Hoyt RW. Individualized Avatars with Complete Anatomy Constructed from the ANSUR II 3-D Anthropometric Database. Intl J Dig Hum. 2017;1(4):389-411. https://www.researchgate.net/publication/317270225_Individualised_avatars_with_complete_anatomy_constructed_from_the_ANSUR_II_3-D_anthropometric_database
10 Pickle N, Zehnbauer T, Harrand V, Zientara GP, Zhou X, Roos P. Avatar Animation for Warfighter Mission Simulation - A Fully Automated Software for Animation of 3D Medical Avatars for Warfighter Mission Simulation. J Trauma Acute Care Surg. 2021;91(S2):S107-S112. https://pubmed.ncbi.nlm.nih.gov/34117168/
11 Woods B. From head to heart to diet, AI is learning to make a map of elite athletes’ bodies. CNBC online. Published Dec 9, 2023. https://www-cnbc-com.cdn.ampproject.org/c/s/www.cnbc.com/amp/2023/12/09/amazon-microsoft-ai-is-training-olympians-and-nfl-players.html.
12 O’Donovan MP, Hancock CL, Coyne ME, Racicot K, Goodwin GA. Assessing the Impact of Dismounted Infantry Small Unit Proficiency of Quantitative Measures of Collective Military Performance Part 1: Recommended Test Methodologies. Natick, MA: U.S. Army Combat Capabilities Development Command Soldier Center; 2023.
13 South T. These are the new pieces of wearable tech coming to the Army in 2024. Army Times. Published Dec 25, 2023. https://www.armytimes.com/news/your-army/2023/12/25/these-are-the-new-pieces-of-wearable-tech-coming-to-the-army-in-2024/.
14 Brown SAT, DeSimone LL, Burke TM. Blue light exposure effects on sleep attributes in a 72-h training exercise. Proc Hum Fact Ergo Soc Ann Mtg. 2021;64(1):971-975. doi: 10.1177/10711813206412
15 U.S. Department of Defense - Responsible Artificial Intelligence Strategy and Implementation Pathway. Prepared by the DoD Responsible AI Working Council in accordance with the memorandum issued by Deputy Secretary of Defense Kathleen Hicks on May 26, 2021. Washington, DC: Dept of Def; 2022. https://www.ai.mil/docs/RAI_Strategy_and_Implementation_Pathway_6-21-22.pdf
16 Guertin N. (2022). Digital Twin Assessment, Agile Verification Processes, and Virtualization Technology. Washington, DC: Dept of Def Director Operational Test and Evaluation. 2022. https://www.dote.osd.mil/Portals/97/pub/reports/(U)%20Digital%20Twin%20Agile%20Verification%20and%20Virtualization%20Technology%20Report.pdf?ver=Gk7mtRbW8lOiFqLisQsmMg%3D%3D
17 Allen G. Understanding AI Technology A concise, practical, and readable overview of Artificial Intelligence and Machine Learning technology designed for non-technical managers, officers, and executives. Washington, DC: Dept of Def Joint AI Center;2020 Apr 1;2(1):24-32. https://www.ai.mil/docs/Understanding%20AI%20Technology.pdf
18 Hull L, Sevdalis N. Advances in Teaching and Assessing Nontechnical Skills. Surgical Clinics of North America, 2015;95(4):869-884. doi: 10.1016/j.suc.2015.04.003
19 Leuze C, Zoellner A, Schmidt A, Fischer M, Cushing RE, Joltes K, Zientara GP. Augmented Reality Visualization Tool for the Future of Combat Casualty Care. J Trauma Acute Care Surg. 2021;91(S2):S40-S45. https://pubmed.ncbi.nlm.nih.gov/33938509/
20 Zientara GP. Second Sight: Vision From A Brand New Perspective. Combat & Casualty Care. 2023;(August):20-21. https://tacticaldefensemedia.com/wp-content/uploads/2023/09/CCC-Aug2023-WebPDF.pdf_1694201
21 Kosourova E, “Data Science Glossary: Definitions for Common Data Science Terms.” May, 2022. Accessed on 19 March 2024 at https://www.datacamp.com/blog/data-science-glossary
22 Hodson DD. Performance analysis of live-virtual-constructive and distributed virtual simulations: Defining requirements in terms of temporal consistency. Air Force Institute of Technology; 2009.
23 Wentworth D, Behson SJ. Implementing a new student evaluation of teaching system using the Kotter change model. High Educ Stud. 2018;45(3):1-13. doi: 10.1080/03075079.2018.1544234.
24 Welke KF, Diggs BS, Karamlou K. Chance, Bias, and Confounding: Threats to Valid Measurement of Quality. Ped Card Surg Ann Sem Thor Cardiovasc Surg. 2010;13(1):79-83. doi: 10.1053/j.pcsu.2010.01.003.